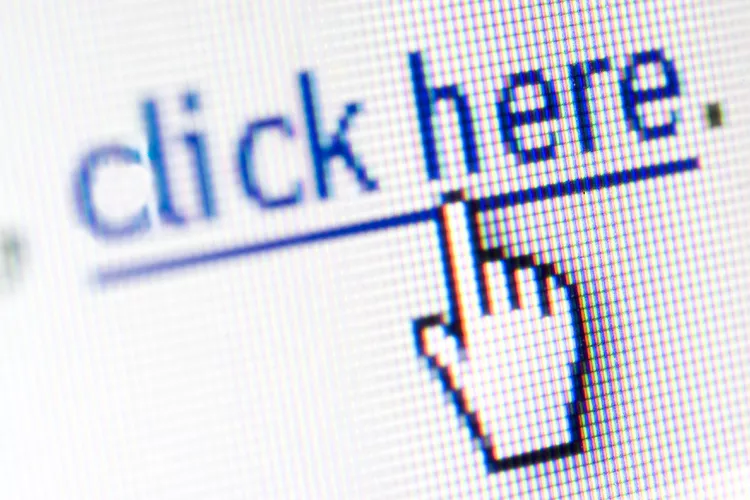
Assorted links for Friday, April 12:
- Software Supply Chain Attestation the Easy Way
- How Many Is Too Much? Exploring Costs of Coordination During Outages
- How We Keep Our Government Apps Running With High Reliability: A Peek at Our Incident Management Strategy
- Infrastructure as Code is Not the Answer!
- 20 Terraform Best Practices to Improve your TF workflow
- SBoMs with Dracon, cheaper, faster, more accurate, better
- The State of Secrets Sprawl 2023
- The COGS Problem
- Presets for bazelrc
- The Biggest Cloud Native Strategy Mistake